by Jan Yorrick Dietrich
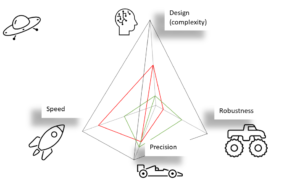
Figure 1: Four dimensions of a nice vehicle. The red volume might be a formula one car while the green volume might represent a rally car.
Have you ever asked yourself why you cannot have a vehicle that is as fast as a rocket, as agile as a formula one car and as robust as a monster truck at the same time? The rocket might be lightning fast, though it will probably fail on tight bends. The formula one car may be quite fast and agile, though it will probably break down quite fast if not carefully driven on asphalt. Well, and a monster truck might be very robust, though it may be slow in comparison. In many fields of research, scientists encounter the same issues as described above. Every new development comes with certain downsides. If you try to satisfy every need, you might get a Jack of all trades, master of none.
The vehicle of my dreams should be fast, precise, robust, and nicely designed – all at once. A rally car is quite fast, though it is slower than the formula one car or a rocket. They are both similar in precision, though the rally car is a lot more robust than the formula one car. Lastly, I personally like the design of a formula one car a lot more. The vehicle of my dreams might fill the grey pyramid itself, being perfect in every dimension.
Leaving the car analogy
This article is supposed to be on the recent advances and future visions in the field of optical measurement processes and artificial intelligence (AI). So how does this go together?
Before I get into more detail here, I want to make sure that we are all on the same page: The history of measurements dates back as far as 9000 Years, as first peoples used the human feet in order to measure distances. Since then, we have come a long way, utilizing not only mechanical measurement devices such as rulers, but also types of measurement that use the knowledge of physics and chemistry to develop smart measurement devices. In short, an optical measurement makes use of light and its properties to measure distances, inconsistencies and roughness, to name but a few.
If you want to measure a complex geometry, you will deal with the same tetrahedron of process variables, shown earlier in Figure 1 with focus on the car analogy. You can choose between multiple methods of measurement, some might be extremely fast and accurate, though they are sensitive to vibrations, some other technology might be robust and accurate, with the downside of being a lot slower. This is quite easy to understand if we try to get an idea of the scales that play into this. Some optical measurement devices are accurate down to nanometers, which is one million times smaller than a millimeter. A vibration inside a production environment might not be detected by the human body e.g. by touching something, but that does not mean that they don’t exist – they are just so small that they can only be detected with very sensitive devices.
In the end, physical principals limit some technologies at the complexity of the geometry an object may have. You can think about it like this: If I was to take a picture of the back of your head, I have no idea how your face looks like – but if you stand in front of a mirror, I might be able to glimpse both.
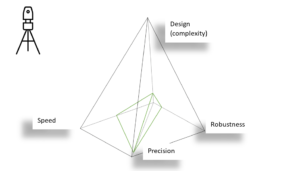
Figure 2: General need description of optical measurements in four dimensions. The green volume may represent interferometry.
Every four dimensions combined can then be displayed with the tetrahedron of optical measurement:
The green volume in Figure 2 illustrates the interferometric principle. Generally spoken, interferometry is very precise (measured in nanometers), quite fast (measured in milliseconds), but also sensitive to vibrations. Those can usually be eliminated in laboratory environments but not in production processes. Therefore, robustness might be quantified in a non-scalar dimension that starts at Laboratory environments in the center of the tetrahedron and goes towards production environments at the edge. Eventually, interferometry is also limited with regards to the design of an object – the geometric complexity.
Now you might ask yourself, why we cannot have it all at once. Considering the car analogy, this might look trivial to you, though with focus on the area of optical measurement, many uncertainties arise, the faster, more accurate or resilient you need to measure. Measurements technologies are often limited by physics of quantum processes and the rules of optics – with the Heisenberg uncertainty principle1 being one of those phenomena.
The new Idea
For now, engineers tend to optimize the components of a system one by one, like the sensor or the processing unit, which can improve the system to a certain extent but this won’t allow us to optimize a system to its full potential. Traditionally, engineers need a lot of experience and testing for any complex measurement task, as we are unable to develop the system all at once.
This is where COMet, Computational Optical Metrology2 enters the stage: Scientists of the University of Bremen are currently developing a new method that utilizes artificial intelligence to optimize those measurements in its entirety. To do so, the group of researchers originate from multiple fields of research, including mathematicians, physicists, and computer scientists.
To understand the COMet concept, I want to take you on a short trip through the basics of optical measurements.
What is inside?
Any traditional measurement system contains a sensor, e.g. a camera that receives information. This information can be analyzed afterwards by a computer and the data that was fetched can be interpreted. This interpretation is called “solving the inverse problem”, as we can only measure effects and thereby investigate the causes. You can imagine this by how we measure and analyze earthquakes (effects) to learn more about the causes inside our planet.
One type of systems, on which the team focused to improve, are interferometric measurement systems. Even though the word itself sounds difficult, you might even know the basic concept. In school, you might have heard that light can both have properties of a particle (photon) and a wave. In some respects, electromagnetic waves behave very similar to water waves – they can stack or even eliminate themselves, as shown in the famous double-slit experiment, which is demonstrated in Video 1.
Video 1: The double slit experiment. When shooting particles at a double slit, they arrange in an interference pattern on the wall behind the double slit. This can be explained, when (light) particles also have properties of a wave. Recommended to watch until 11:35 min.
The patterns that occur will represent a certain superposition of two waves, which is just a fancy way of saying, how they overlap. To keep it simple: Those measurement systems make use of this phenomenon and can thereby give almost perfectly accurate measurements. Unfortunately, this method can only give insight of the superposition of two waves, but it cannot give information on how far anything is in absolute terms. The reason for that is that we can only measure the offset of two wave crests (as we know the wavelength of our measurement) by analyzing the patterns, but we don’t know how many amplitudes (amount of wave crests) are between the sensor and the object we want to measure. Those kinds of issues only get harder to imagine the deeper we dig into the details of optical measurements.
Fortunately, we do not always have to know every detail. For example, knowing the offset might sometimes be everything you want to know. When checking for small bumps on a relatively flat surface of a product, it might be totally fine to know the offset with high precision, as you are not interested in how far the surface is actually away from the sensor – all you want to know is the roughness of the surface. So even if our measurement lacks certain types of information, we might be very satisfied with the results. At the end, every measurement technique comes with a different set of pros and cons.
For now, we have covered a lot of theory, this is why I want to give you some real-world applications in the following.
What smartphones have to do with optical measurement
Another way of optimizing a system is to save cost on devices, especially expensive optics. Let us have a look on our smartphone cameras, as they are a quite common optical measurement devices of our daily use. Manufacturers usually install small (and cheap) optics, which lead to image defects if they are not processed afterwards. By means of computational imaging, pictures can be optimized, as developers teach the smartphones in which way the camera is not ideal, to enhance picture quality. You can imagine this like adapting a filter onto your picture, that makes it look better. The problem with this is: Those ‘filters’ only have a good fit for certain surroundings and may not be ideal for every lighting situation etc. as they cannot adapt to those perfectly. An example of this is shown in Figure 3.
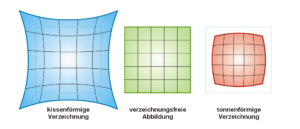
Figure 3: Distortion of images: This is desired in the case of fisheye lenses, but with conventional cameras distorted images must be corrected by mathematical tricks3
Where the magic happens
Bremen researchers came up with the idea to utilize artificial intelligence to take this idea to the next level. After a COMet system got information about the measurement apparatus and the measurement object and data was fetched, data will be analyzed with respect to the parameters of the measurement system. This analysis will come up with new optimized parameters for the measurement, which are then sent back to the system. The next measurement, adapting the new optimized set of parameters can then be analyzed again. This cycle may be repeated several times, to optimize the system even further with every run.
The analysis of all parameters can be stored and translated to a logic language, which an artificial intelligence can understand and utilize. The archived information can even combine to different sets of information. Scientists call this process knowledge representation and reasoning. Lastly, the more data you put in, the better and more reliant forecasts and optimizations will be created by the AI by means of deep learning techniques.
If everything works out as Bremen scientists think, they can imagine tools that will be able to (partially) plan and execute new measurements with little input.
Therefore, the researchers call for a paradigm shift, like the one of compressed sensing, which led to a vast number of new algorithms and changed the way how data was fetched and processed in the mid-2000s. (The car analogy to this is about hydrogen and electric engines, in contrast to combustion engines)
Quick and easy
At the beginning of this article, I introduced you to the vehicle of my dreams, which had to fulfill four aspects, all at once. We quickly got a feeling for the problems that scientists encounter with almost any new development, the fact that some dimensions are simply contradictory to others, and that we can not have it all at once.
Making the jump to optical measurement, I think it is safe to say that if we keep investigating the performance of single components, further enhancements will come to an end rather fast. Computational optical metrology is just at the beginning, though we can imagine that utilizing knowledge about physical processes and powerful tools as AI and compressed sensing to its fullest potential will improve the measurements of tomorrow within multiple dimensions, including cost and time effectiveness. Many fields of research have already adapted methods of artificial intelligence very effectively with language recognition being one of the most famous applications. Products like “Alexa”, “Hey Google” and “Siri” would not understand a thing if they were not using the powerful tools of AI. Assisted driving also is a field of AI research. If adopted correctly, those systems have the potential to improve driving safety a lot in combination to human driving. Bremen researchers hope to utilize similar synergies as not only researchers in the field of engineering and computer science, but also physicists and mathematicians work together at this new field of research.
1. Further reading: The Heisenberg uncertainty principle, explained by The Guardian
↩
2. Bergmann, R B; Falldorf, C; Dekorsy, A; Bockelmann, C; Beetz, M; Fischer, A
Ganzheitliche optische Messtechnik
Quelle: Physik Journal 18, 2 (2019) 34-39
3.
https://de.wikipedia.org/wiki/Verzeichnung#/media/Datei:Verzeichnung3.png
Leave a Reply