Blogpost written by Vishnu Mohan and Gopi Krishnan
Key Points
- Analysing the self-regulatory process of complex science learning
- potential and opportunities of artificial intelligence (AI) methods to analyse self-reporting protocols for recognizing cognitive and metacognitive strategies of self-regulated learning.
- Efficiency of human classifier and AI classifier
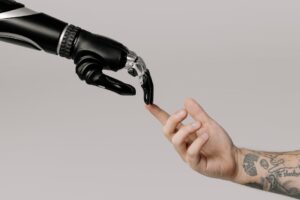
Are you frustrated about the progress your tasks projects and studies, don’t worry an AI powered model is being designed to analyse Self-regulatory learning and its strategies. Self-regulated learning is a cyclical process, wherein the student plans for a task, monitors their performance, and then reflects on the outcome (Zimmermann). Self-regulated learning helps individuals to drive their learning car through a right track. It is evident that learners those who failed to be in resonance with Self-Regulated Learning could benefit less from their learning journey.
Cognitive strategies are a combination of active learning technologies for example summarizing information, enhancing comprehension and retention. As well as Meta cognitive strategies focus on self-awareness and regulation. Allowing individuals to monitor their own learning process. In collective, these strategies create a powerful working model to improve self-regulated learning it is clear that the proper analysis of self-regulatory learning Enhance one’s learning experience. The learning is entered into digital era the emphasis should also go to self-regulated digital learning. It’s clear that employing self-regulated learning strategies will facilitate learning autonomy.
It is found that recognizing cognitive and metacognitive started this of self-regulated learning through conventional methods is time consuming as well as impossible to an extent.
Here a study done by C-Y Wang and John J.H Lin Is important as well as relevant done with the to investigate the potential and opportunities of an artificial intelligence mother to analyse self-reporting protocols for recognizing cognitive and metacognitive strategies of self-regulatory learning
Scope of the study
In this digital era, usage of artificial intelligence in self-regulatory learning is popular. If a machine learning system is able to extract cognitive and meta cognitive an activity from a complex data set will make the analysis easier. Do artificial intelligence already became one of the most influential technologies in digital learning here we are standing with an argument well-functioning AI based classification will be helpful
The study
Aim of this study is crystal clear and understandable as well as that will definitely drag our attention to the result. Researchers explore the scope of an artificial intelligence model to classify Cognitive and metacognitive moves in complex science tasks. Rather than a research or study it’s an effort to build a bidirectional artificial intelligence powered Long-Term Short-Term Memory (recurrent neural network (RNN) in which cells are connected along a temporal sequence) to classify cognitive or metacognitive activities implemented and self-reported by the learners during the task-solving process.
In a nutshell, C.-Y. Wang, John J.H. Lin conducted this study to in this study, we aim to explore the potential and opportunities of utilizing an AI model to support the identification of cognitive and metacognitive activities from verbatim protocols of scientific explanations evaluation tasks in a Self-regulatory learning context
The fact that human coders need a variety of domain knowledge to analyse cognitive or metacognitive information increases the feasibility of an artificial intelligence model, and that a human-cantered perspective can help an artificial intelligence model provide information for decision-making can be considered as fuels for this study.
Along with exploring the scope of an AI model researchers specifically emphasized on (1) how the way the AI is built (its structure) and the dropout rate used during training affect how well it works or performs its tasks. (2) To what extent are the judgements between human and AISE consistent? (3) What differences can occur between human-based methods and AISE, and where do these differences potentially come from?
Methods
To achieve our goal, a Long-Term Short-Term Memory model called AISE was designed to recognize cognitive and metacognitive moves from long narratives. The whole procedure in implementing AISE consists of five stages, namely data collection for the SRL task, data labelling, data preprocessing, implementation of AISE, and evaluation of AISE. 39 Undergraduate students from forums of social media in northern Taivan selected as participants of the study. After the above-mentioned stages different statistical test and a qualitative analysis conducted to have solutions on above mentioned specific concerns
Results
After such a scientific and statistical methods and analysis, it’s found that potential of AI model and some points of improvement to be done.
The specific emphasize of the study brought a very clear results the study says that machine learning is capable of classifying learners Cognitive and meta cognitive strategies from thinking process protocols, but one critical think which must understand is sample size of the study was limited. But statistical data and analysis shown a fair consistency between humans and AISE. Moreover, the application of AISE own similar task but with different topics also shown a very good performance.
Beyond these, we found that the accuracy of the LSTM model is affected by complex text patterns containing both temporal cues and extended segments involving multiple cognitive and metacognitive actions ie co-occurring or overlaid cognitive and metacognitive activities in a paragraph.
Conclusion
This study sum ups with a hope that an AI model can work fairly well to support analysing self – regulated learning, that is self-reporting protocols for cognitive and meta cognitive strategies. In our view the discrepancies founded can be treated with more structured and educated machine system.
As well as this research broadens the doors for further research about how AI models can perform more effectively with long verbatim, text, dataset. Even though this research explored the scope of AI in analysis of Self-regulated learning still discussed urges on this topic. After reading this paper, we are thinking about how an automatically periodic feedback system can amplify self-regulatory learning. Which will help learners and researchers to review their process.
References
Wang & Lin (2023): Utilizing artificial intelligence to support analyzing self-regulated learning: A preliminary mixed-methods evaluation from a human-centered perspective. https://doi.org/10.1016/j.chb.2023.107721
Huang, S., & Wang, H. (2016). Bi-lstm neural networks for Chinese grammatical error diagnosis. dec (Osaka, Japan).
Image:https://www.pexels.com/photo/bionic-hand-and-human-hand-finger-pointing-6153354/
Papamitsiou, Z., & Economides, A. A. (2019). Exploring autonomous learning capacity from a self-regulated learning perspective using learning analytics. British Journal of Educational Technology, 50(6), 3138–3155. https://doi.org/10.1111/bjet.12747
Zimmerman, B. J. (2002). Becoming a self-regulated learner: An overview. Theory into Practice, 41(2), 64–70.